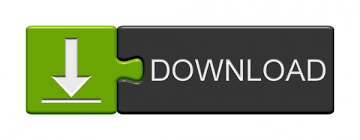
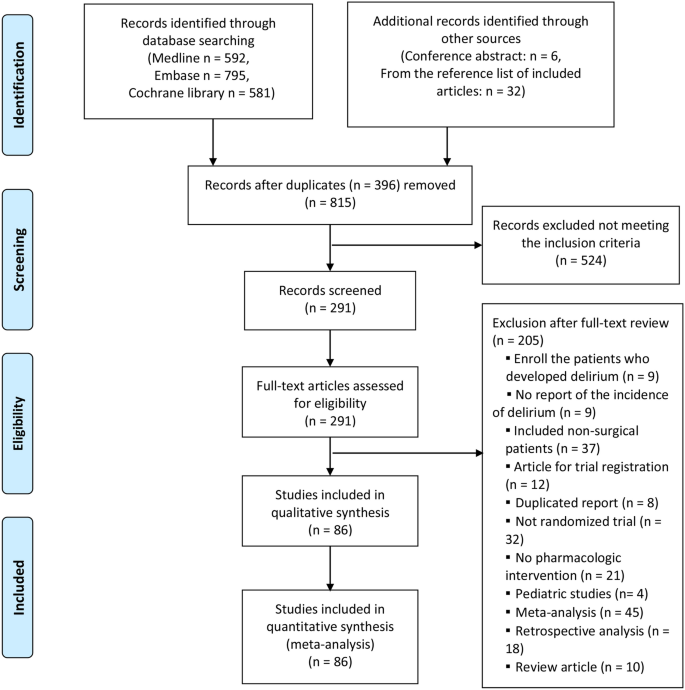
The quality of both levels defined in PLS-SEM analysis, i.e., the measurement model and the structural model, were assessed on performance measures defined in literature. Validation was done through partial least squares structural equation modelling (PLS-SEM).
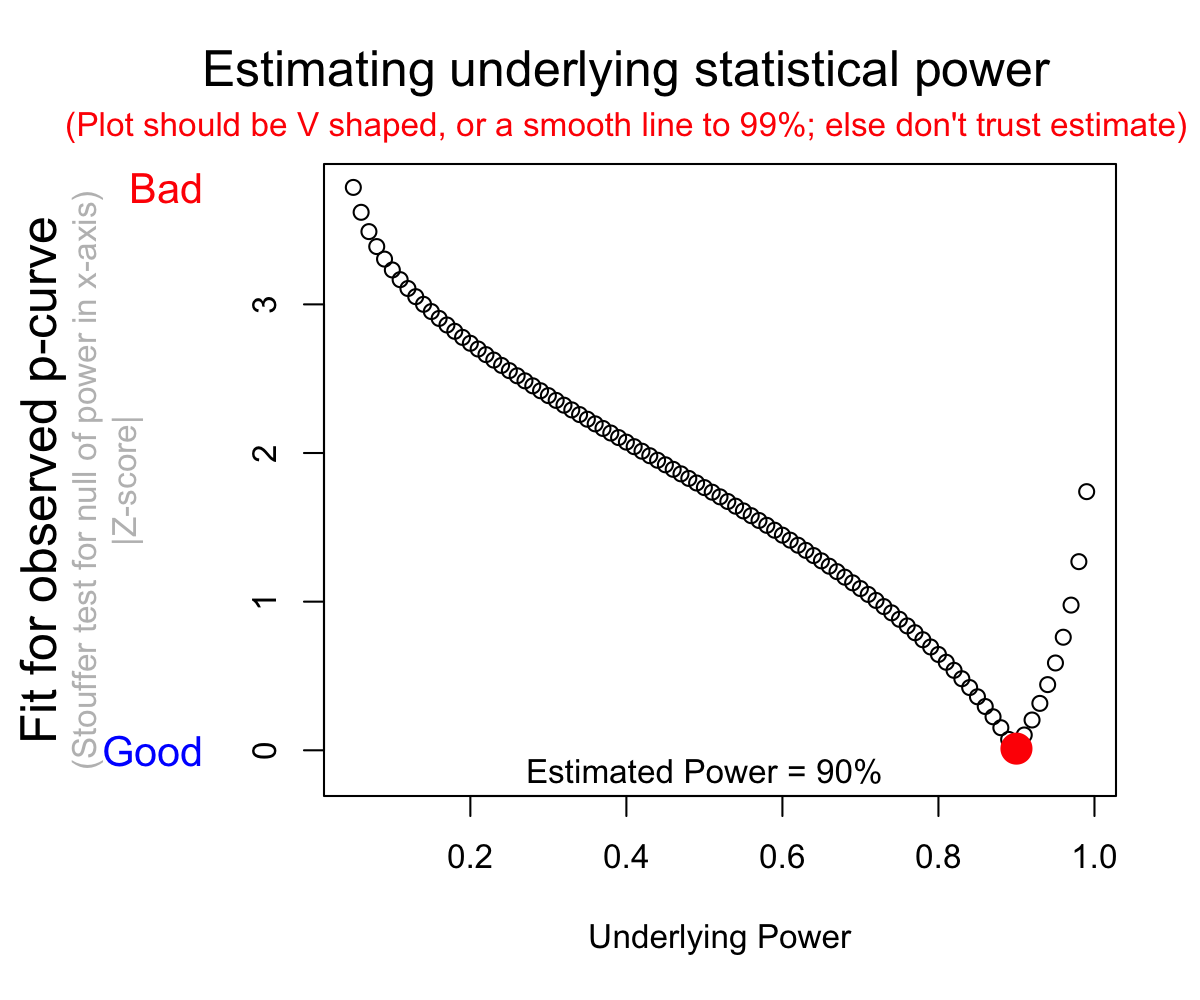
Using a questionnaire built upon this model we gathered data to perform a summative validation of our model. Methods: Based on separate models from the literature we developed a new theoretical model describing the underlying concepts of the adoption of structured and standardised recording. For a RVSOM model, these conditions cannot be met, as the variance shift applies to a single observation and we However, their results assumed either that the data values were independent and identically distributed, or that the data set could be partitioned into a number of independent subsets such that the number of subsets increased with the size of the data set. Stram and Lee showed that the asymptotic null distribution of the LRT for testing this type of hypothesis is a 50:50 mixture of two chi-squared distributions on zero and one degree of freedom. This is because the null hypothesis falls on the boundary of the parameter space and regularity conditions are not met. Although the restricted log-likelihood is suitable for constructing likelihood ratio tests for var- iance components provided the mean structure of the null and alternative models are the same, the stan- dard asymptotic theory which relates the distribution of LRT statistic to a c 2 distribution under the null does not apply here. LRT j = 2 (5) The LRT statistic is analytically intractable except in special cases, but readily obtained from standard mixed model software. Both Baker and Jackson (2008) and Lee and Thompson (2008) used the fl uoride toothpaste dataset that is re-analysed here Lee and Thompson used Markov Chain Monte Carlo to overcome the dif fi culties associated with integrating out the random effect. This distribution comprises two halves of a t-distribution with differing scale factors, ‘ glued together ’ at the mode so that the probability density function and its fi rst derivative are continuous (Fernandez and Steel, 1998). Lee and Thompson (2008) explored the use of the skewed t-distribution in a Bayesian context. This means that that their numerical methods are quite liable to be fragile in repeated routine application. A dif fi culty with their methodology is that by using maximum likelihood estimation in conjunction with non-tractable random effects distributions, the classical methods proposed by Baker and Jackson (2008) required both numerical integration and numerical maximisation. Baker and Jackson (2008) explored the use of several non-normal random-effects distributions, of which the t-distribution performed best however, they did not examine skew distributions. There has been little work on fi tting non-normal distributions for the random effect in meta- analysis.
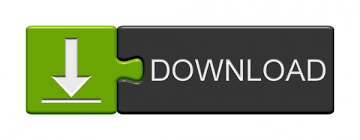